[January 10, 2025]
Autonomy for the World: MQM-178 Firejet
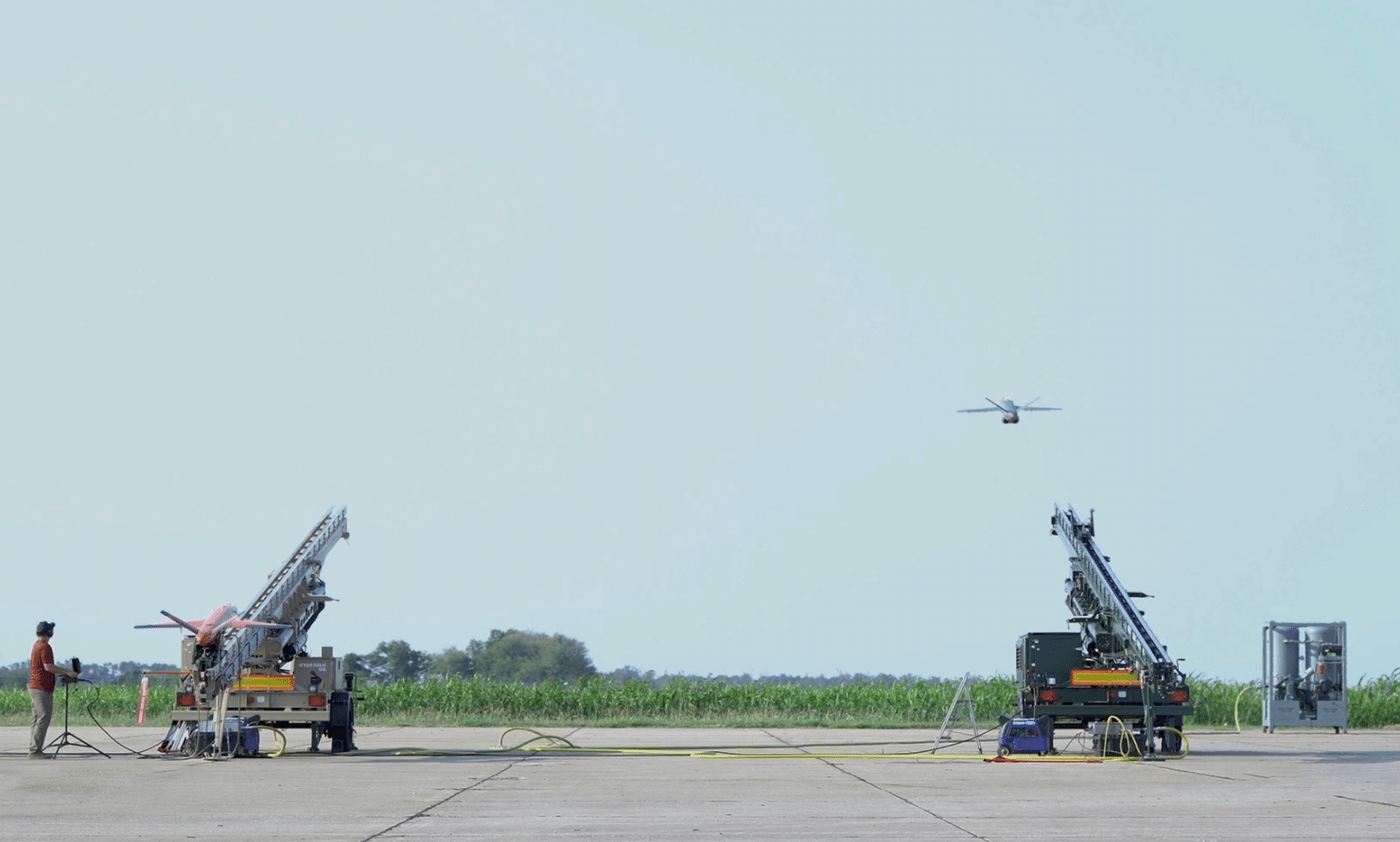
At Shield AI, we’re pushing the limits of human-machine collaboration, advancing Autonomous Collaborative Platforms (ACPs) to work seamlessly with piloted aircraft. Our recent tests with jet-powered drones demonstrated autonomous formation flying and tactical maneuvers, laying the groundwork for integrated operations between crewed and uncrewed systems—an essential step for the future of multi-domain air dominance.
In July 2024, Shield AI conducted dual-ship autonomy tests using Kratos’ MQM-178 Firejet drones near the Kratos facility in Oklahoma. These tests represented a shift from single-aircraft autonomy demos to multi-agent with human-machine operation, essential for the autonomy needed in future operational environments.
During these tests, the Federal Aviation Administration (FAA) required that each Firejet be accompanied by a safety chase plane for visual oversight, creating a unique four-ship, collaborative human-machine setup. With two unmanned jets flying over 300 mph alongside two manned jets in restricted airspace, trust in the AI’s ability to operate autonomously becomes critical. While not ideal for specific test case operational testing, the inclusion of safety chase pilots introduced requirements and capabilities very similar to manned-unmanned teaming scenarios.
Historically, in manned-unmanned missions, the human pilot has managed collision avoidance. For true collaborative operations, however, drones must identify these risk situations and perform these maneuvers independently, without relying on a human-in-the-loop. While still providing the manned pilots with enough awareness to maintain trust and safe operation. The autonomous platform to platform coordination and deconfliction is a critical element of scaling any future capability. This project marked the latest advancement in applying Shield AI’s Hivemind product to uncrewed systems and capped a phased series of tests designed to integrate Hivemind on third-party platforms.
Building the Foundations
Given the risks associated, we conducted our flight objectives with a crawl, walk, and sprint methodology. Thus, allowing us to identify and mitigate technical and operational risks in a timely manner. Our initial flights centered on validating the Firejets’ control interface and Hivemind’s basic AI functions. Moving from platform setup to flight-ready in 120 days, we achieved the first flight in just 170 days. Following this, we flew every 30-60 days, progressively advancing both the jet hardware and autonomy software, setting the stage for multi-aircraft coordination. Our rapid flight test and software evolution timeline was enabled by our combination of our high-fidelity simulation models, large batch testing leading to frequent flights.
In the initial test, our objective was to validate our AI pilot integration with the existing MQM-178 flight control system. The focus was on confirming control and sensor data interface responsiveness. We ran the AI pilot from the ground control system, sending basic orbit commands to keep the Firejet within a designated area. The initial validation tests were crucial for ensuring that Hivemind could reliably interpret and execute foundational commands, like heading and altitude, without interference or lag. These commands formed the core for later tactical maneuvers, confirming that even under basic conditions, the platform’s autonomy functions as expected.
Onboard Autonomy with Edge Computing
To execute a fully autonomous mission, we installed an edge computer on the Firejets, allowing Hivemind to operate directly on the aircraft. The integration of an edge computer on the Firejets represented a significant adaptation, enabling real-time processing without relying on ground stations. The EC Micro had to handle complex autonomy algorithms and mission calculations independently, marking a major shift from traditional target drone applications to an autonomous collaborative system. Hosting autonomy compute at the “edge” also makes the platform more resilient to communication link outages. Alongside the edge computer, we integrated an encrypted comms-mesh network radio, enabling the Firejets to communicate with each other and with ground control, ensuring cohesive execution of mission objectives.
FAA’s Certificate of Waiver or Authorization (COA) requirements meant we worked within tight airspace restrictions, and safety chase planes provided continuous visual line-of-sight throughout the flights. These added safety measures were essential, given the constraints of restricted airspace. The coordination of safety chase in close quarters with the autonomy agents represented many of the same autonomy agent to human interface and trust scenarios in manned-unmanned teaming missions. These ranged from traditional pre-mission planning to new AI agent “tendencies brief” which conveys expected behaviors under operational scenarios. These new process steps, multiple simulation runs, and real-time system status provided the manned pilots with the needed level of confidence to safely operate with the autonomy agents in the same airspace.
Capstone Flight: Autonomous Formation and Coordination
During the capstone test, each Firejet launched under human control and then switched to autonomous mode once within designated test airspace. Hivemind autonomously piloted the drones through formation, turning, and other tactical maneuvers without human intervention.
We launched the first Firejet on a rail system and handed over to Hivemind upon reaching the test area. The second Firejet, launched in the same sequence, a few minutes behind, joined the first in-formation once it arrived in the test zone. We designed the mission scenarios and commanding issued to Hivemind to be deliberately flexible, enabling the AI to replan in real-time if any obstacles prevented precise formation joins. This flexibility allows Hivemind to respond dynamically to changes, a feature essential for coordinating multiple autonomous agents in a collaborative mission environment. The chase pilots monitored from a safety perspective only; the Firejets flew and coordinated entirely on their own. Each Hivemind agent coordinated with the other and the ground control station while making autonomous decisions to successfully accomplish its mission objectives. The peer-to-peer coordination, agent-ground system awareness, and manned pilot awareness was critical to multi-agent mission.
Dynamic Coordination: Autonomous Decision-Making in Action
Unlike traditional autopilot systems that use waypoint navigation, Hivemind supports dynamic decision-making, allowing the aircraft to actively respond to each other in real time. The Hivemind Cognition Core is designed to provide high level decision making while accounting for mission objectives, needed behaviors and associated actions. For instance, when commanded to “aggregate,” the Firejets autonomously coordinated routes, aligning in formation. Using dynamic rejoin strategies, the second Firejet could intercept the first efficiently, saving fuel and optimizing positioning for subsequent mission phases.
With the chase aircraft’s restrictions on turn rate and altitude climb rate, Hivemind managed formation flight while observing operational constraints. These are mission and global constraints to stay within preset mission and safety limits. During our capstone test, Firejet One detected it was slightly ahead and executed a geometry lag maneuver, allowing Firejet Two to catch up—demonstrating Hivemind’s capability to apply geometry-based adjustments, common in human-led formations, for autonomous alignment. Hivemind’s geometry-based coordination was key in enabling the Firejets to adjust positions relative to each other without explicit human input. These maneuvers, similar to those in human formation flight, allow Hivemind to recognize and execute spatial alignment patterns autonomously, using geometric positioning to anticipate and adjust for optimal formation.
The lag maneuver itself represented many of the advanced capabilities, such as, agent-agent coordination, agent decision making to identify the need for a lag maneuvers, calculating the geometry of the lag resulting in a better formation position, all conducted autonomously. This is a level of autonomy abstracting operator control which allows the operator to focus on mission objectives as if they were flying with a manned pilot in formation.
Advancing Resilient Autonomy for Defense
Our work with Firejet is part of Shield AI’s broader R&D and direct program strategy to accelerate the adoption of autonomous capabilities into new and existing systems for defense applications. By focusing on adaptable, platform-agnostic autonomy, we’re ensuring Hivemind can meet mission needs across a range of platforms and scenarios. With Hivemind, we’re not only advancing autonomy for today’s missions but laying the groundwork for the complex, collaborative operations that will define the future of air combat.
About the Author
Matt Maroofi is a Director of Engineer for System Design at Shield AI, where he leads advanced autonomy and AI integration for global defense applications. With over 20 years in aerospace and defense, Matt has specialized in cutting edge technology advancement in airborne and spaceborne applications, and AI-driven capability integration. Before joining Shield AI, he contributed to key advanced technology projects, holding roles in R&D and operational programs both in industry and government positions. Matt holds a M.S. in information and data science from UC Berkeley, focusing on deep learning and natural language processing, and an M.S. in electrical engineering from George Mason University, specializing in communication and satellite design.
This blog is part of a series of case studies highlighting the unique challenges and accomplishments of integrating Hivemind on each platform it has flown. Each installment delves into the technical innovations, collaborative efforts, and mission successes that define our work and our teams.