[August 14, 2018]
Meet Our Leaders: A Conversation with Andrew Reiter
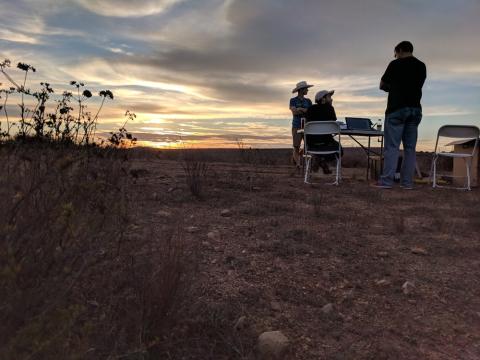
You started your education in chemical engineering. Why did you ultimately pursue a career in AI & robotics?
I had been interested in robotics from a young age, but as I went into college I had a strong interest in chemistry and engineering so I chose to study chemical engineering. As a junior in college, I entered a robotics competition and I really threw myself into it. I spent all my free time on it and was inspired to making our robot better and better with my team.
Ultimately, we ended up winning the competition! I enjoyed it so much I decided robotics is where I wanted to focus. If I hadn’t done that competition, I would probably be a chemical engineer today!
What was it about robotics that captured you?
It’s the ability to make a change, to design something and then immediately see the results. You could program a behavior in the robot, and then watch the robot do what you just told it to do. The immediate feedback that allows you to iterate at a high rate is rewarding.
Since that competition, how have you seen the whole field of robotics evolve and explode?
If we look back at the last 30 years, there’s always been interesting success with laboratory research. What’s exciting now is that robots have become more mainstream and autonomous systems have even entered the home. A toy robot will not just drive around by remote control like it used to be. Now the toys you can buy are flying and autonomous. You can now buy robots that will fly autonomously inside and outside and will recognize you, follow you, respond to you, and you can even speak to them with natural language. It is not just toys, robots are increasingly being used in prosumer and professional applications. It has been really exciting to see all of this become mainstream, not only because I enjoy robots and being surrounded by them, but because it means that the building blocks that we use to build our systems are becoming more and more sophisticated.
When thinking about building a system from the ground up like here at Shield AI, what’s the most exciting and what’s the most daunting?
Pushing the start-of-the-art in software and marrying it to state-of-the-art hardware tailored to this specific mission. Building a quadrotor or any autonomous platform presents a really interesting systems integration challenge, because there are many interdependencies between subsystems. In a quadcopter example, selecting an optimal motor, battery pack, speed controller, computer, and sensor suite is more complex than it may seem. No component can be selected independently.
The software and AI is also complex. The software architecture must balance the competing needs of algorithms for state-estimation, mapping, planning, control, semantic scene understanding, and abstract decision making. Additionally, a great deal of software infrastructure is required for the software development itself as well as data-logging, data-post processing, security, simulation, visualization, test, integration, and deployment. The right abstractions must be incorporated to allow portability to different types of robots.
Understanding and balancing all factors is extremely challenging and also very exciting. It is rewarding to build this system from the ground up
When you think about the whole progression of both state estimation and planning, how do you think about the progression that has been made in this area in the last 5-10 years? Specifically, how does that progress shape what Shield AI is building?
We’ve made huge progress in this arena. By incorporating a lot of advancements that have happened since the creation of our first prototype, we now have highly redundant multi-sensor fusion. We are able to handle any scenario – inside, outside, underground, or in the dark. We can fly at high speeds while keeping precisely on the path that we’ve planned, avoiding obstacles and even avoiding new obstacles that pop up which weren’t there a second ago. We’ve significantly increased the performance of our system, and we’ve been making our own state of the art advancements in those areas ourselves.
It’s almost like being a pioneer if you think about the things you are seeing now that you wouldn’t have thought would be possible when you first began this work, isn’t it?
Yes, and I’ve really noticed the explosion of GPUs and dedicated co-processing subsystems tailored for machine learning – the amount of power we are able to get on our robots and the capabilities that this brings with them is impressive. Early on it was hard to imagine all that we would be able to do onboard, and that we would have this flying robot not just map the world but understand it. Today, it understands where it is, the context of that, what it means for the mission, and how to turn that into something actionable. It begins to ask, what should I do next in order to further the goals of my user?
What are you excited about within electrical, mechanical and embedded strategies for Shield AI?
It’s amazing coming from these early prototype stages where everything is cut carbon fiber sheets, to now,where we have a product that is injection-modeled with custom PCBs. Looking forward, we’re pushing as hard as we can on optimizing that system. On the electrical and embedded side, we’re taking every last off-the-shelf piece we’ve incorporated and replacing that with something custom-built that is very tailored to our needs and optimized to meet those needs in the minimum weight and power possible to maximize our flight time. The same goes for mechanical; we’re investigating new injection-molding state of the art techniques. You wouldn’t be able to assemble an equally capable prototype in a lab and have it fly for more than five minutes, but by pushing the state-of-the-art in production manufacturing, in plastics, in our own PCB design and integration, we’re able to take that platform that would otherwise fly for five minutes and fly it for more than 20.
For new people joining the team, what do you think is something exciting about the work being done here?
We are working on a new way of representing the world, that really unifies all of the different areas in which we are working. Rather than having one representation of the world that is optimal for state estimation, and another optimal for mapping and another optimal for object detection, and yet another optimal for obstacle avoidance – we are working on this exciting new technique that unifies all these different areas such that sensor information can directly go into a consistent, underlying representation that is true to the nature of the world and is accessible to all analysis we might like to perform on it. This is a big deal.