[January 15, 2019]
Shield AI Fundamentals: On The Challenges of Autonomy
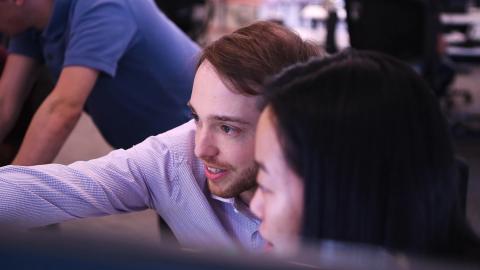
A conversation with Andrew Reiter, Shield AI’s Co-Founder and Technical Fellow.
How is indoor autonomy different than traditional autonomy? What makes indoor autonomy so challenging?
When it comes to unmanned aerial vehicles (UAVs), autonomy has traditionally referred to the ability of a plane to fly without needing a pilot (remote or otherwise) at the stick. There are escalating levels of autonomy, from simple stabilization that keeps the UAV airborne, to waypoint guidance & control that gets from Point A to Point B, to advanced behaviors that choose their own destinations in order to achieve some higher-level goal such as sensor coverage of an area.
Flying indoors presents a host of new challenges. GPS is not available, so simply figuring out the robot’s location is a nontrivial task. The environment is uncontrolled. It may present unique challenges for the robot’s set of sensors, algorithms and actuators, with items such as hanging light fixtures, furniture, and other household clutter that the robot must navigate.
What problems have you had to solve that are unique to indoor autonomy? How does Nova overcome these challenges?
We faced many unique challenges while designing Nova to be successful indoors — especially since we want Nova to be successful outdoors as well. From a high level, the biggest challenge is in selecting sensors that will function in all conditions. GPS does not work inside, cameras don’t help when it’s dark, and LIDAR fails in environments that lack sufficient unique structure. Nova uses all three — plus another dozen sensors — to ensure that there is always some modality that is providing sufficient information. We have designed custom algorithms that are able to fuse information from all sensors, implicitly deciding which sensors can be trusted or not at any given time, and exactly how much to trust them. Our algorithms allow for seamless indoor-outdoor transitions, elegantly handling periods in which the set of useful sensors is changing rapidly.
There are a number of specific challenges we’ve had to address that are typically not encountered outdoors. LIDARs have trouble with dust, so we’ve selected models that handle dust better than others, and have added software filtering that looks for the unique signature of dust and removes it from the data. Glass and mirrors are difficult to detect optically, so we’ve added acoustic sensors, and look for the sort of reflections that glass tends to produce. High thrust in narrow hallways can generate vortices that reduce lift, so we’ve made our system as lightweight as possible to keep these vortices to a minimum.
What separates Nova from other autonomous robots, including, the Roomba vacuum cleaner?
Most robotic vacuum cleaners simply bumble about our living rooms in a combination of spiral and random patterns. They require very little knowledge — just reactive obstacle avoidance, and, given enough time, they will probabilistically cover your whole floor. Some of the more advanced models will try to map out your walls and furniture as they go so they can ensure full coverage.
Nova not only maps out the buildings that it enters and seeks full coverage, but it does so at high speed, following time-optimal flight trajectories. It flies up and over obstacles, clearing whole buildings in just a few minutes. It streams live video to an operator, annotating objects of interest as it goes. Although the two robots share one behavior in common, the similarities end there.