[December 4, 2018]
Shield AI Fundamentals: On Progress in Swarming
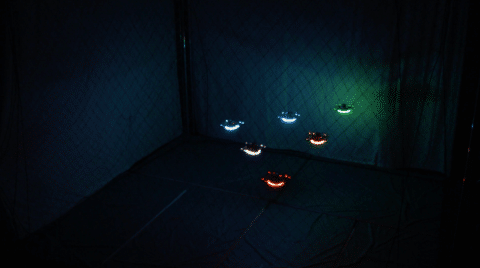
A conversation with Professor Nathan Michael, Shield AI’s Chief Technology Officer. This is a continuation of our conversation of Shield AI Fundamentals: On Multi-Robot Collaboration.
Is the idea of swarming decades away? How do you answer the question of when swarming will be possible in the real world?
There are different levels of what is possible, from possible in the lab to possible in the field as reliable products. We actually know how to do much of what is required from a theory perspective and just need to take action to address the engineering challenges that arise when designing and developing reliable and capable swarming systems.
I envision three to five robots in the field having non-trivial impact within two years. From there, moving to swarms of approximately 10 robots not that long afterward, with even larger 100 robot swarms shortly thereafter; this rapid advancement is possible due to the theory and ideas that have been richly developed over the last 40 years. The unsolved parts are becoming fewer and fewer. We actually know how to do much of this now.
How do you think about going from five robots in the field to 50 or 100? What do you view as the biggest challenge?
The greatest challenge is the variability that can arise as a consequence of the environment; each robot’s performance will change along with their ability to interact depending on the evolving conditions. We previously deployed between 30 to 50 robots persistently over many power cycles within a laboratory environment where these systems continuously operate and achieve desired tasks while interacting with each other. A key observation in this type of controlled environment is that tremendous complexity arises as each robot’s ability to operate changes rapidly over extended use. These same observations are commonly noted in large-scale deployments such as warehouse package delivery, where systems can change substantially across runs (e.g., wheels wear down, battery capacity degrades). However, the domains that we consider at Shield AI exhibit characteristics that are substantially more complex with conditions that are not readily anticipated.
In the lab, we observe that failures manifest at the individual robot level pretty quickly, in the first few runs. But, after they are running for a much longer period of time, then it starts to becomes a question of reliability; which is more a question of robust systems engineering and less a question of algorithm design and correctness. As such, the primary challenge scaling from 5 to 10 robots is largely algorithmic and scaling from 10 to 50 robots becomes less algorithmic and more a question of engineering. From 50 to 100 robots and higher, there tend to be fundamental changes we make to algorithms. But, it’s important to note, the fundamental changes are less substantive than the challenges of achieving reliable performance across large numbers of systems. That becomes more an engineering challenge than a theory challenge.
You have spent decades in the laboratory wrestling with these questions. Is it unique or interesting for you to see more people asking these questions as the ideas of multi-robot collaboration and swarming have become more mainstream?
There is a certain uniqueness to it in how much the perspective has changed over the last 10 to 15 years in terms of what is possible. I would say that the evolution has been really rapid, but at the same time, unsurprising.
A lot of the past challenges come down to algorithms and theory that you are developing over time, but which were gated by engineering and technology. Now that engineering and technology have reached a place where you can see a lot of these theories brought into the real world with impact, then a lot of these algorithms are ready to be applied; the theory and the engineering and technology are aligning in terms of maturity. So I am not surprised at all and I expect it to continue to progress quickly as there is 30 to 40 years of backlog of theory just waiting for the right moment in time when technology catches up — and that time is now.
How do you think about the idea of robots learning from each other and becoming smarter?
It’s the same way that we as people learn from each other and that is through experience. We all learn about the world and then we share those experiences with each other. That’s the way the robots get smarter.
The challenge is developing the algorithms that permit that kind of approach — algorithms that make it possible for a robot to experience and to operate in the world as it explores an environment, perceive and model that environment, and then take that experience and make it possible to share it with other robots.
We’ve seen some examples of that in your videos where the robots are learning from each other how to avoid certain obstacles. Was it a significant moment for you when you first observed that robots could learn from each other in the lab?
It was certainly interesting to see, but this occurred as part of an engineered system, so it was also unsurprising. It wasn’t this a-ha moment because it didn’t do anything magical; the system did exactly what it was supposed to do. I suppose the fact that it did exactly what it was supposed to do — that the robot learned how to generate the knowledge and experience and then transfer its knowledge to other robots that were much smaller and unable to compute the same types of solutions — was exciting. But it also was unsurprising because the theory says it is supposed to work, and that it should provide these types of guarantees of correctness, and that it will work.
What was really interesting was what occurred after that point, because now the systems could develop experience that we could not generate as well. The more the robots flew, the more capable they became. They flew faster, they became more resilient and more capable of handling increasingly complex and challenging operational contexts. So this was the original starting point that we needed in order to create truly high performing systems — we needed to be able to enable those systems to think for themselves and therefore drive their performance higher. Again, it is very interesting, but also unsurprising to see that that’s what happened because that is what the theory said was supposed to happen. They flew faster, they become more resilient, and they became more capable of handling increasingly complex and challenging operational contexts.
What is the role of resilience in swarms?
Resilience in swarms refers to the ability of the system to mitigate unanticipated challenges. This is achieved through the system’s ability to figure out that something is wrong and then adapt to the issue that may be causing problems in the system, accruing insight over time (through experience and using many types of learning techniques, such as reinforcement learning) to improve individual and collective performance.
Resilience refers to the ability to deal with this very large number of permutations of errors that can arise when fielding robots in the thousands. You can create resilience through clever systems design, but ultimately, because of the complexity of the design, you end up having to create algorithms that allow the systems to coordinate and collaborate in a way that the robots can really identify those issues as they arise and adapt to those issues by making decisions; planning together; sharing information; choosing to listen or not listen to other robots depending on whether they are making mistakes; and how they allocate resources or task robots based on their ability to contribute to the operating conditions. So resilience manifests at many different levels in a hierarchical structure from the lowest level of an individual robot to the highest level of groups of robots working together to figure out how they can deal with changes in the operating conditions and their expected levels of performance.