How did research concerning multi-agent and multi-robot collaboration become one of the central thrusts of your academic career?
I began working with multi-robot collaboration and coordination through my Ph.D. effort. Working with an advisor, I focused my work on teams of robots and how we think about designing feedback control strategies to drive a team of robots to follow a particular path or work together in a certain way, and in a manner that is scalable.
Why did you choose this area?
I was interested in this idea of arriving at collective intelligence, sharing knowledge, overcoming fundamental challenges in communication and coordination. In society, we regularly have to deal with the challenges that come with having groups of people work together. The same types of problems emerge in robotics. I wasn’t fundamentally driven to study multi-robot collaboration exclusively; I was more driven by this idea of how do we pursue self-directed autonomy and how do we do that through individual and collective intelligence.
After I completed my dissertation, I took a step back to focus on the question of how we pursue fundamental concepts related to individual intelligence and introduce resilience. How do we expand that back to collective intelligence? And then I brought those ideas of resilient intelligence to collective intelligence and coordinated systems.
At that time, was this a path many other researchers were pursuing?
These ideas on collective intelligence were circulating, but the landscape and the era were quite different compared to today. You pursued a path in which you combined research areas with a focus on collective intelligence.
At that time when I was completing my dissertation, we were showing results with teams of 5, 8, 10 robots, and that was significant. In terms of my path, I have pushed in a number of research directions concurrently, and the rationale for that push was that, although these different areas and the concurrent pursuit of these areas meant we had to do more and engage in much more extensive research, it also meant we could bring the ideas into a more unified whole. So instead of just existing in one particular area of expertise, and then having to accept what’s going on in state of the art in other areas, if we pushed and defined the state of the art in a number of areas, building upon my work and the efforts of the broader research community in recent decades, we could drive that work together and forge a more cohesive whole. This pursuit of the state of the art across several areas enabled us to truly pursue these more challenging questions of resilient collective intelligence.
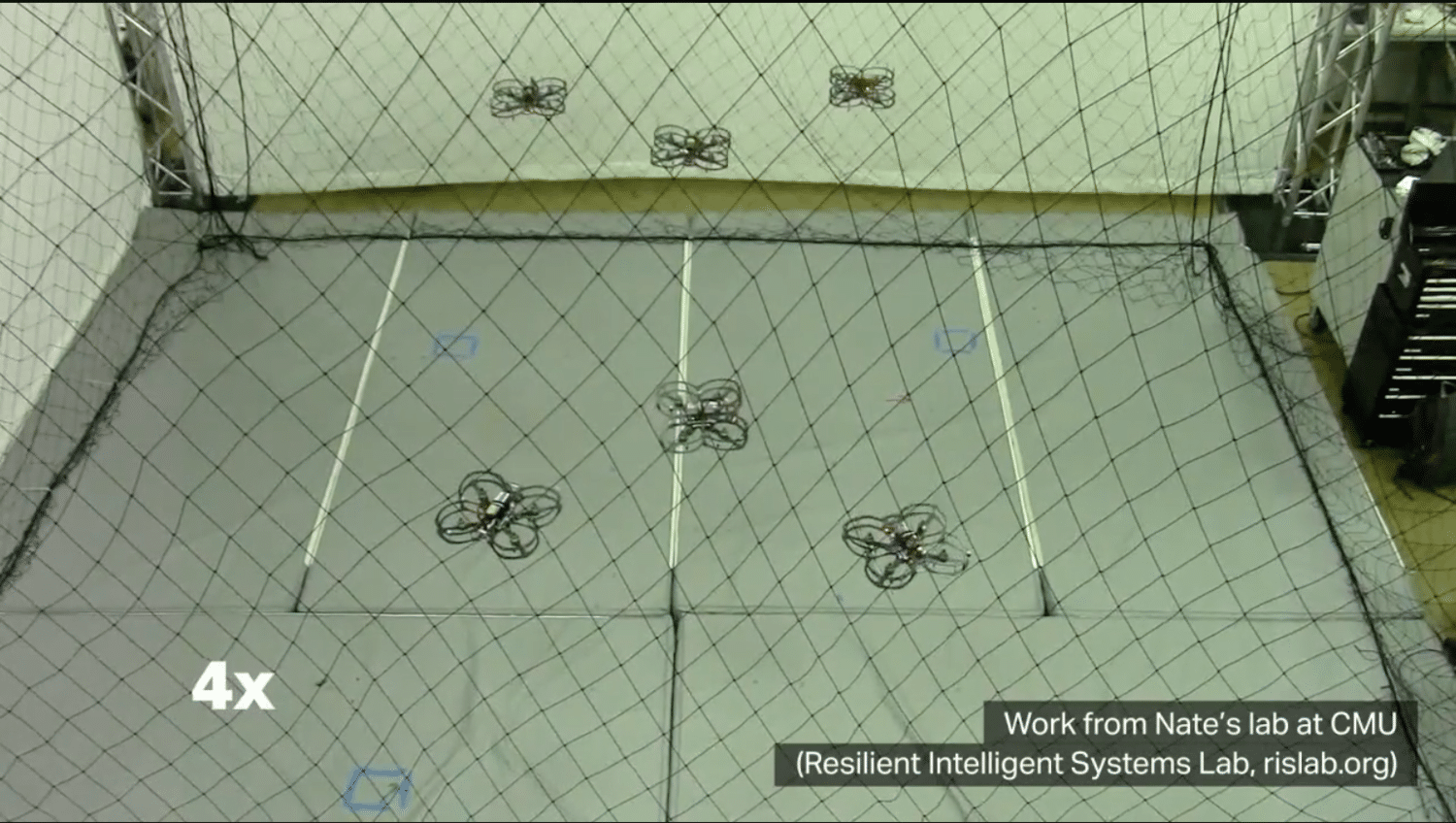
How do you think about the state-of-the-art today compared to when you first undertook this work?
The distance that we have traveled is significant in terms of advancement. A decade ago we were excited if we could have 8 to 10 robots fly in a formation and do so quickly. Or 20 robots move quickly through different shapes. Now, we have 50 robots flying constantly, taking off, landing, recharging, and completing different tasks. They may be forming different formations, or operating in different types of domains where the operating conditions are variable.
We do this over long periods of time, and, as the robots learn about their performance both individually and collectively, some robots perform well, and others perform worse. The system itself learns how the robots should be deployed in order to overcome both individual and collective limitations while still achieving the goals of the operations. And in the event of an individual retasking the system, the system and the robots think about how that request changes what they want to do and how they can best achieve the intent of the person while simultaneously ensuring safety and feasibility.
A decade ago we were laying the foundation for this work. And as you lay one brick and then another brick, you start to rise in terms of how high you can actually go and the sophistication of your algorithms and the sophistication of your methodology. As we laid those foundations then, building upon our work and the work of the broader research community, we started to think about not just how individual robots could work as a single system, but how they could move together, how they could observe and monitor the world around them individually, and then collectively. How they could make decisions as a team as they move together in order to change what they’re doing in order to achieve a goal. And how they could think about this not just over the instantaneous or the next few seconds, but then over longer time horizons taking into account their own individual limitations and their ability to execute. That allowed us to begin to actually model how a person engages with the system, how to understand their intent in the context of the manner in which the robots operate, and the way that they perceive and execute tasks. And this work and this learning just keeps building upon itself. Eventually you reach the point where you have built a system that is really able to adapt to challenges, to learn from failures, and to keep going with really limited degradation in performance even in profoundly dynamic circumstances.
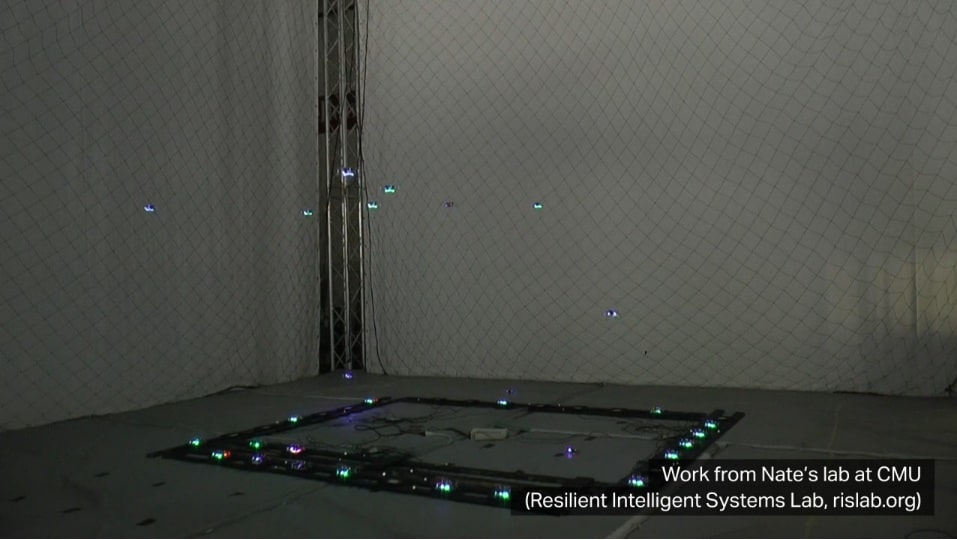
What are some applications of collective resilient intelligence that can make a difference today and tomorrow?
I can give you a specific representative problem. Let’s think about a robot that has to drive or fly through a crowded environment where there are a lot of people walking around. The robot needs to be able to understand how those obstacles are moving and then make a decision of where it should go in order to avoid obstacles while at the same time making some progress toward some goal.
Now imagine that it is not a single robot, but a team of robots we are talking about, and that team of robots needs to successfully navigate this crowded environment while making certain to remain in a particular formation so that it can continue to operate as expected. That’s a general scenario that can be applicable in monitoring or responding to a disaster event or a scenario in which you have a large number of people moving through a domain. It could arise within the context of self-driving vehicles as they are moving through a cluttered urban environment. This problem of single and multi-robot coordination while driving though dynamic obstacles is a very hard problem because the robots have to think about how they move and where they move, and they also have to think about how the environment is changing and how these changes impact the decisions they make. Additionally, if the environment changes, the robots have to change their decisions locally, and they have to therefore change their decisions collectively as well.
What you end up reaching is this really computationally expensive problem in which, unless you’re able to really have the robots think and perceive the world in the same mathematical space that they are also planning in, it’s very difficult for them to do their work quickly while responding to rapid changes in their dynamic environment with, for example, people or cars moving quickly. These are exactly the kinds of problems we focus on at Shield AI, building upon the unified approach to collective resilient intelligence whose foundations were laid by my lab and the broader research community over the last several decades.