How do you think of trust as it pertains to the operation of an AI system?
The concept of trust in an AI system is similar to how you trust that when you turn on your car, because it’s in park, the car won’t move. If you turned on your car, and all of a sudden the car started lunging forward, your trust of that engineered system would go down substantially.
We trust that an AI system will work as we expect it to because it’s based in the theory and the underlying principles that drive forward this technology. From a science and engineering perspective, when we create artificially intelligent systems, they are just engineered systems. However, these systems become so complex that it’s difficult for us to really understand their inner workings. Trust starts to come into play, from a human perspective, when we are engaging with systems that are sufficiently complex that we cannot know, and so we have to trust, that they will operate as they are expected to.
How do you build trust in the AI system’s operation?
In order to be able to understand how these types of AI systems operate, you have to look at the different technologies that are used. There are many different forms of AI and, depending on which type of machine learning or robotics you are looking at, there are different ways of assessing the metrics of performance. One of the major areas where trust starts to manifest is in the role of accuracy and correctness of what these systems are learning, and whether or not they’re learning “the right thing.”
AI systems use data-driven techniques to draw conclusions. So, being “right” or “correct” is entirely based on interpretation of data in order to draw conclusions. When an AI system learns the wrong thing, it’s wrong because its conclusion doesn’t match what we would expect. At the same time, it’s “right” in that the AI system is learning what it should based upon its programming and the data going into it.
Building and verifying trust with these types of systems is really all about quantifying and analyzing the data. We need to quantify the types of data that we’re using — the breadth and depth of that data, the information the AI is receiving, the AI’s conclusions — and use that information to determine how the accuracy of the system is impacted in different scenarios. This helps us to understand what we’re actually learning from this underlying system.
Why is trust an important factor in building AI systems?
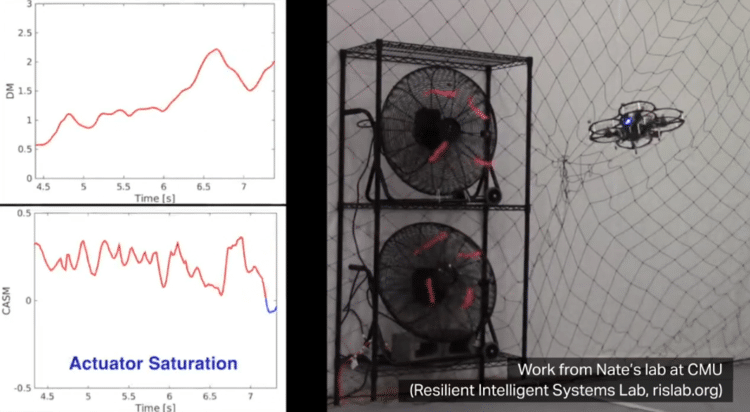
Trust is important for the same reason that it is important that we build bridges that do not fall down in expected operating conditions. When we are building AI systems, these are just engineered systems that operate within certain expected conditions and to specific requirements. When we build these complex engineered systems, every subsystem and component within those systems must all adhere to certain requirements or specifications. We establish requirements such as an accuracy threshold or the ability to operate in specific conditions. By holding to those particular requirements, the components, as you put them together, start to compound. That compounding relationship is then considered. As is the relationship between how each of these constituent components impact each other.
In the same way that we build any engineered system, we build AI systems to engage with expected levels of performance in terms of accuracy, quality, consistency, reliability, and robustness. All of these are measures that we look at to make sure that these systems are hitting certain margins. If they aren’t above those margins, then those systems cannot be trusted. They’re not ready to be used. They’re too immature, so we’ll spend more time maturing them. Additionally, when we start to combine these systems, how those subsystems interact creates additional complexity. It creates additional areas for interaction and additional modes of failure, so we must assess that as well.
How is the performance of those subsystems assessed?
It is assessed in the same way as any other complex engineered system: through a lot of careful, statistical analysis. We look at large sets of data, over large test scenarios, over large numbers of trials, under many different environments and performance characteristics and demands upon the system. We observe how those systems perform, and we assess the performance characteristics both in simulation and in real world experience. We use this type of performance assessment to understand the reliability and performance characteristics of the system. Ultimately, reliability is the measure, just like any other engineered system, that tells us whether or not we can trust that our system works as expected.