[August 19, 2018]
What is State Estimation in Robotics?
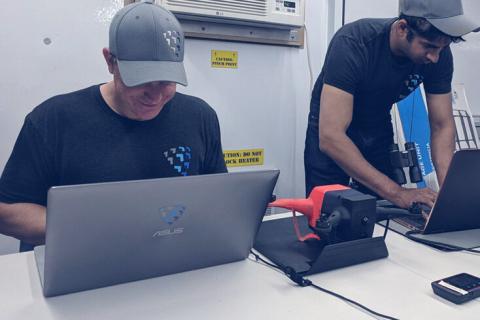
What excites you about the work you do with state estimation at Shield AI?
Pushing the envelope. GPS-denied navigation and mapping have been an active research topic for years. Researchers have made great strides, and, building on top of that work, we are able to demonstrate really compelling autonomous capabilities. Of course, there is still much to be done. We are striving to achieve truly anytime, anywhere navigation and mapping capabilities.
The first challenge is robustness; there is a really big difference between demonstrating a capability and making it work each and every time. While that doesn’t sound like a very glamorous challenge, it is actually quite rewarding because of its scope and scale. Applying small patches or fixes is not a viable solution; you inevitably end up with a growing number of fixes which require ever-increasing amounts of fine-tuning to maintain and often end up in conflict with one another. This means that a principled approach is required. Whenever we encounter a failure mode, we go back to fundamentals, ask “what assumption was violated?”, “how was our model incorrect?”, and then we adapt our solution accordingly. This requires a deep understanding of the algorithms and results in their evolution.
The next challenge: information discrepancies. These can be broken into fundamental deficiencies (e.g. trying to navigate in the dark relying on a visible light sensor) and latent information (the information we need is embedded in the sensor data, but not readily available). To address fundamental deficiencies, we investigate new sensing technologies. which is actually a very exciting field right now, driven by the gaming, automotive and drone industries. To mine latent information, we look to cross-discipline approaches. For example, machine learning techniques have gotten very good at recognizing places and inferring information about 3-D geometry from a single (or just a few) images.
Why is state estimation so important?
I think you could count state estimation and mapping among the real cornerstones of mobile autonomy. Knowing where the robot is and understanding its surroundings are fundamental requirements for virtually any autonomous capability. Our group takes pride in striving to make reliable state estimation and mapping something other groups can take for granted.
What made you choose this startup?
I was at the point in my career where I was beginning to understand that in order to make a difference and to do something meaningful, I was going to have to take a risk. What made Shield AI particularly appealing were three characteristics: a mission that matters to me, exciting work in emerging technologies and the high potential for real success
Who or what has been the most influential on your career?
My ultimate cause is my family. They have always encouraged and supported me and tolerated a lot so that I could do what I do. My advisors gave me confidence and something to aspire to. When I was an undergraduate, I worked as an intern with a group of really exceptional people at Honeywell, Inc. Much that I have accomplished I would never even have attempted if they had not made me feel like I belonged among them. My graduate advisor is the person I try to emulate most in what I do to this day. He is one of the brightest, most capable and most respected people that I know, but he still has deep humility and genuine compassion for others. My inspiration comes from my colleagues and the leadership at Shield AI. You can’t help but be at your very best when you are surrounded by this much talent, passion and dedication.
You’ve been at Shield AI for years now. What is your favorite Shield AI memory?
Early on, almost two years ago now, we sent a team to a two-day demo. The customer’s reaction was something I’ve now come to expect after every demo, but about which I never tire of hearing: having seen our robot in action, it hits home that this is a real capability, not theoretical. They now see things that had been really cool ideas associated with some indeterminate future transformed into immediate, real-life possibilities. In this case, after the first day, the sponsors envisioned a particular add-on capability and asked if we could demonstrate it in a video the following day using the data from the first day. They had asked for a capability that we had been working on but wasn’t ready yet, so the demo team said “we’ll try!” A couple of other engineers and I stayed up all night working to finish implementing the capability and create the video. I think of this event as capturing the heart of technology development at Shield AI. We don’t always work all night, and gratification doesn’t always come in a single day, but Shield AI epitomizes the ideology that the reward is proportional to the effort. I’ve worked hard before, for great companies, but that next day was the first time I felt like I had really had an impact. I think that feeling combined with an important and meaningful mission is what gives the team at Shield AI so much passion for what we do.